‘Diagnosis of Basic Learning Skills Task Battery’ Modified for Engineering Students
Abstract
Background. The higher education system today requires students to be able to conduct independent academic work outside the educational system. Some data has been developed on the general educational skills of students; however, the available works are most often devoted to the skills of students in the humanities, yet in technical fields such as engineering, scientific knowledge becomes outdated much faster, which is associated with the global digitalization of society.
Objective. To assess the Diagnosis of Basic Learning Skills Task Battery (Metodika diagnostiki osnovnykh uchebnykh umenii) as modified for engineering students.
Design. The study was conducted in several stages. First, we created six sets of tasks for assessment of basic learning skills, based on the subject matter of engineering disciplines for students at three educational stages (first-year students, fourth-year students and second-year master students). Next, engineering students at different educational stages at Moscow Technological University (N = 135) took part in testing of the proposed task battery. They were also administered the Diagnosis of Supplementary Learning Skills Inventory by Ilyasov (questions for self-assessment), and a survey of academic performance and socio-demographic variables. Skills of memorization and consolidation of knowledge were not assessed in the current study.
Results. Confirmatory factor analysis allowed us to establish high convergent validity of the task battery (p = 0.001). Internal consistency of the separate scales of the battery was acceptable (Cronbach’s aranged from 0.692 to 0.839). Тhere were significant positive connections between the modified task battery for diagnosis of basic learning skills and the battery for diagnosis of supplementary learning skills, academic performance, and educational stages.
Conclusion. The results demonstrate that the modified battery is a valid and reliable tool for measuring basic learning skills.
Received: 22.12.2019
Accepted: 07.08.2020
Themes: Educational psychology
PDF: http://psychologyinrussia.com/volumes/pdf/2020_3/Psychology_3_2020_145-160_Ilyasov.pdf
Pages: 145-160
DOI: 10.11621/pir.2020.0310
Keywords: educational psychology, learning skills, engineering students, task battery, convergent validity
Introduction
Composition and Properties of the Learning Skills
The modern system of university education in the Russian Federation is characterized by an increased ratio of unaided, self-sustained student activity in acquisition of subject knowledge and skills. The reason is the transition to the European educational model, characterized by increasing social and professional mobility of under- and postgraduates, conversion to a system of continuous and dual education, and the need to improve graduates’ competitiveness. The main goal of the ongoing modernization of the higher educational system is to develop the student’s educational independence. The formation of learning skills, the universal ability to carry out educational activity unrelated to its subject matter, has a particular influence on the development of that independence (Hattie, Biggs, & Purdie, 2013; Mamaril, Li, Usher, Economy, & Kennedy, 2016; Mitrofanova & Simonyan, 2016, Marra , Kim, Plumb, Hacker, & Bossaller, 2017; Uden & Dix, 2004).
The organization of this activity for students is associated with the increasing role of the concept of Lifelong Learning (Butenko et al., 2017; Knapper & Cropley, 2000). The ability to learn throughout life is crucial for engineering students, as they must treat their careers and skill sets as dynamic and permanent update required (ABET, Inc., 2013; Smerdon, 1996).
There are two groups of researchers studying learning skills. The first group understands them as the capacity for metacognition. These skills include memory, thinking, and intelligence through schematization of concepts, learning strategies, self-reflection in learning, motivation, and control (Feuerstein, Rand, Hoffman, & Miller, 1980; Flavell, 1987; Zimmerman, 2002). The second group adheres to the competence approach, according to which the primary role in the ability to learn belongs to the needs of society. For them, the ability to learn is characterized by competent behavior, which includes a cognitive component, control of affect and socialization, general educational and subject skills (Hautamaki et al., 2002; Raven & Stephenson, 2001).
It is important to note some commonality among these concepts. In general, learning skills can be subdivided into the basic skillsof acquisition, construction, and consolidation of subject knowledge in the student’s experience, and supplementary skills, as a conscious processing of psychological and pedagogical factors affecting basic learning skills (Ilyasov & Simonyan, 2018).
From this point of view, we rely for our theoretical background on Ilyasov’s concept of the composition and properties of learning skills (see, e.g., Graf, Ilyasov, & Lyaudis, 1981; Ilyasov et al., 1984; Ilyasov, 1986; Ilyasov, 2016; Ilyasov & Simonyan, 2018; Lyaudis, Ilyasov, Malskaya, & Mozharovskii, 1989).
According to Ilyasov’s concept, the process of constructing knowledge consists of perception, logical and creative thinking, and the understanding of speech. These can be carried out dependently (based on information provided), independently, or by combining dependent and independent types of knowledge construction (Ilyasov et al., 1984). Learning skills are cognitive and mnemonic processes and their development, along with the assimilation of subject knowledge in various fields of science and practice, is the essential task of any training program (Khutorskoy, 2003; Kraevsky, 2003; Marra et al., 2017). Cognitive and mnemonic processes are in turn linked with such psychological functions as motivation and will, emotions, and self-awareness, influencing cognition as a whole (Gordeeva & Sichev, 2017; Hautamaki et al., 2002; Ilyasov, 2016; Leontiev & Klein, 2017; Raven & Stephenson, 2001).
The development of supplementary learning skills can be described as a reflective influence on psychological and pedagogical factors in the learning process. Those skills develop through the increase of a person’s awareness of their qualities, abilities, and environmental characteristics (Griffin, 2018).
Students’ academic performance may depend only on more than self-regulation, metacognition, and motivation. Some authors have found that less than half of first-year engineering students end up graduating in engineering (National Academy of Sciences, 2005). Predictors of engineers’ academic success include: high school GPA, quantitative skills (ACT, SAT, or GRE), and math scores (Veenstra, Dey, & Herrin, 2008). These can be used as criteria for admission to university, where the students can acquire basic learning skills.
The development of basic learning skills can be represented as the change and increasing sophistication of those skills throughout the learning process. This development takes place continuously in the process of interaction with the student’s environment, both subjective and social, and is primarily spontaneous and non-reflective. Often this happens in the process of subject learning at its various stages (Ilyasov & Kostrova, 2017). The combination of reflective and non-reflective factors in the process of subject learning underlies the construction and development of learning skills.
Diagnosis of the cognitive components of learning skills is carried out in many countries when students enter universities as undergraduate and graduate students (SAT, GRE, PISA). However, the data obtained are not considered in terms of the development of abilities, with changes by learning stage and age; the evaluations are more likely to be subject-specific (Griffin, 2018; Sternberg, 2004).
There is some data on the development of students’ general learning skills; however, the available works are most often devoted to the skills of students in the humanities, yet in technical fields such as engineering, scientific knowledge becomes outdated much faster, which is associated with the global digitalization of society.
In Russia, there is no commonly adapted tool for diagnosing students’ cognitive skills (general learning skills), but an instrument for diagnosing basic (and supplementary) learning skills in the humanities was developed by Ilyasov (2016).
However, the curricula of the humanities and engineering disciplines are naturally quite different. In the humanities, there are courses in knowledge, cognition, teaching and learning (such as philosophy, logic, psychology, pedagogy). The curriculum of engineering courses, on the other hand, includes no courses on cognition other than philosophy, and there are many technical disciplines that are expected to develop students’ analytical and logical abilities.
This article presents a modification of Ilyasov’s Diagnosis of Basic Learning Skills Task Battery, designed by Aslanova for engineering students in different courses. We present the initial results of the testing and structural validation of this task battery, intended for research and practical purposes.
Methods
Content of the Validated Material
The research used two methods of diagnosing learning skills, one for basic and one for supplementary skills assessment. We also collected personal data (gender, age, year of study) and the academic performance scores of the respondents. The latter was represented by mean scores (academic ratings), rounded to the whole according to mathematical rules to simplify further analysis.
The method for basic learning skills diagnosis was based on technical disciplines, according to the curricula of engineering students. There were six variants of the task battery, each containing 25 tasks to assess students’ abilities to construct knowledge in various ways (see Appendix A). We did not include the consolidation of knowledge at this stage of the research.
The first part of the battery tested students’ abilities to construct knowledge provided to them in finished form (dependent knowledge). It included five tasks to check their information-decoding abilities, such as perception of the sign form of the elements; actualization of the meaning of linguistic units; reproduction of content; the contextual meaning of words and the ability to distinguish the meaning of words and to use them correctly.
The second part consisted of 11 tasks to diagnose the skills of independent knowledge construction by induction, deduction, and hypothesis formation (using comparison, discrimination, identification, etc.). To do that, the participants had to use their previously acquired subject knowledge.
The third part of the task battery was diagnosing knowledge construction skills involving both dependent and independent methods. This part included eight tasks on the ability to determine the thematic composition, structure, and layout of a text with its graphic image, characteristics of phenomena, types of knowledge presented, etc.
Approbation was made on the student samples belonging to the three stages of learning: first-year students, fourth-year students and second-year master students (Table 1).
Table 1
Correspondence of the stages of learning
|
First stage (first-year students) |
||||
|
Second stage (fourth-year students) |
||||
|
Specialists: Third stage (second-year master students) |
||||
Bachelors |
1st course |
Specialists |
1st course |
Specialists at MSU |
1st course |
2nd course |
2nd course |
2nd course |
|||
3rd course |
3rd course |
3rd course |
|||
4th course |
4th course |
4th course |
|||
Graduated |
5th course |
5th course |
|||
Masters |
1st year |
Graduated |
6th course |
||
2nd year |
Graduated |
The Diagnosis of Supplementary Learning Skills Inventory is a self-reporting questionnaire measuring students’ level of reflection on their ability to utilize factors of learning (Ilyasov, 2016). The inventory consists of 26 questions for self-assessment related to studying at the university (“When studying at the university, I …”), with possible answers “never”, “rarely”, “often”, and “always”, on a Likert-like scale from 0 to 3 points. The following scales were distinguished:
-
Students’ processing of psychological factors of learning, with the following subscales: impact on motivation and will; consideration of one’s own cognitive abilities; emotional state regulation; time management while performing educational tasks;
-
Students’ processing of pedagogical factors of learning, including overcoming learning obstacles.
Processing of the training process was not included in the current research.
Participants
The total sample (N = 135) comprised students from technical universities in Moscow, 65.9% men and 34.1% women, aged from 18 to 33 years (M = 21.6, SD = 3.2). Among them, 37.8% were first-year students, 37% were fourth-year students, 25.2% were second-year master students and graduates.
Procedure
Respondents completed the tasks mainly ingroups. Participants from the first-year studentsgroup were tested in the second semester of the 2017–2018 academic year and received a pen-and-paper variant of the tasks. Students from both senior groups were tested in the first semester of the 2018–2019 academic year and received computerized variants of the task battery.
The following hypotheses were tested:
-
There are direct positive correlations between basic and supplementary learning skills.
-
Students’ academic performance is positively linked to the level of their basic learning skills.
-
Students in first-year courses have a low level of basic learning skills.
Results
Factor Structure of the Task Battery
The factor structure of the task battery was checked to analyze convergent validity. We also performed a reliability test of the scales based on the tasks with the highest factor loadings and a correlation analysis.
The main criterion for validation was the student’s academic performance. Relationships were established between the scales of the proposed modified task batteryand the indicators of supplementary learning skills and level of education.
A confirmatory factor analysis was performed using IBM SPSS Amos software, during which we relied on the initial assumption of a 3-factor structure of the method. The factors were:
-
Dependent construction of knowledge;
-
Independent construction of knowledge;
-
Mixed construction of knowledge, combining both variants above.
Then, due to the results of the analysis, the original theoretical model was transformed to increase the validity (see Figure 1).
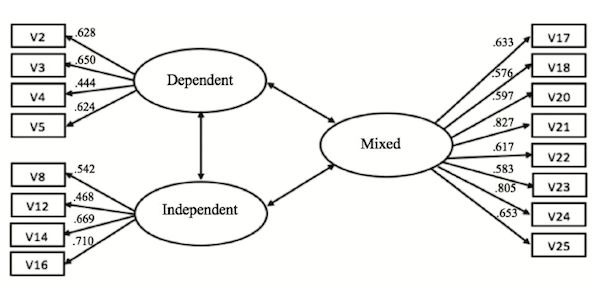
Figure 1. Parameters of the structural model of the Diagnosis of Basic Learning Skills Task Battery scales (N = 135)
Factor loadings of the tasks, indicating high empirical validity of the model are shown in Figure 1. The factors were consistent with the original theoretical scales, but had a lower degree of consistency, so the decision was made to reduce the number of tasks in each factor. This reduction significantly improved the parameters of the structural model (Table 2).
Table 2
Model Fit Summary
Model |
CFI |
RMSEA |
Degrees of freedom |
Chi-square |
NFI |
AIC |
Default model |
.931 |
.058 |
87 |
125.777 |
.819 |
255.777 |
Note. CFI = Comparative Fit Index; RMSEA = Root Mean Square Error of Approximation; NFI = Normed Fit Index; AIC = Akaike’s Information Criterion
Internal Consistency of the Scales
The scores’ distribution on the scales was normal or close to normal. The asymmetry values ranged from -0.21 to -0.78, the excess was from -0.78 to 0.52.
The internal consistency of the tasks on the scales was acceptable, so the scales needed no further changes. As expected, with the general sample, the scales showed high positive correlations with each other (measured by Spearman correlation coefficient; significant at p < .01) (Table 3).
Table 3
Internal correlations (Spearman correlation coefficient) and descriptive statistics for the scales with the total sample (N = 135)
Scale |
Dependent construction of knowledge |
Independent construction of knowledge |
Mixed construction of knowledge
|
Dependent construction of knowledge
|
1 |
.426**
|
.420** |
Independent construction of knowledge |
|
1 |
.664** |
Mixed construction of knowledge
|
|
|
1 |
Average value |
7.32 |
5.92 |
10.62 |
Standard deviation |
2.52 |
2.05 |
5.98 |
Asymmetry |
-0.787 |
-0.374 |
-0.215 |
Excess |
0.592 |
-0.023 |
-0.787 |
Cronbach’s a |
0.692 |
0.719 |
0.839 |
Note. ** p < .01
Those correlations were repeated when the general sample was split into three parts.
Relationships Between the Scales of the ‘Diagnosis of Basic Learning Skills Task Battery’ and Students’ Academic Performance
Analysis of the relationships of the task battery scales with students’ academic performance identified significant correlations (Table 4).
Table 4
Correlation between scales of the test battery and academic performance
Scale of the method |
Dependent construction of knowledge |
Independent construction of knowledge |
Mixed construction of knowledge
|
Academic performance |
.253** |
.277** |
.282** |
Note. ** p < .01
The students were then divided into three subgroups (low, average, high), according to the diagnosis of their basic learning skills, using cluster analysis (K-means clustering). An assessment of the differences between clusters (by the Kruskal-Wallis test) confirmed their significance (Table 5).
Table 5
Sample clustering according to the level of basic learning skills (Kruskal-Wallis)
|
Low |
Average |
High |
Significance of differences (Kruskal-Wallis H-test)
|
Dependent construction of knowledge
|
5.43 |
7.93 |
8.41 |
25.527 p = 0.000
|
Independent construction of knowledge |
3.97 |
6.36 |
7.41 |
51.056 p = 0.000
|
Mixed construction of knowledge
|
2.95 |
11.39 |
17.85 |
113.281 p = 0.000
|
N |
37 |
61 |
34 |
|
Based on the results of the cluster analysis, it was possible to assess the distribution frequency of various levels of academic performance (ratings) by the levels of their basic learning skills (Table 6).
Table 6
Cross-tabulations of differences in learning skills with academic performance
|
Level of basic learning skills |
|||
Low |
Average |
High |
||
Academic rating |
3 |
45.0% |
40.0% |
15.0% |
4 |
28.3% |
54.3% |
17.4% |
|
5 |
22.7% |
42.4% |
34.8% |
The table shows that students whose average rating was close to the “satisfactory” mark (3) mostly demonstrated low (45%) or average (40%) basic learning skills. Students with the rating “good” (4) generally had average learning skills (54.3%). Students with an average rating close to “excellent” (5) showed average and high basic learning skills.
Relationships Between the ‘Diagnosis of Basic Learning Skills Task Battery’ and the ‘Diagnosis of Supplementary Learning Skills Inventory’
The correlational analysis (Spearman correlation coefficient) between the scales of the Diagnosis of Basic Learning Skills Task Battery and the Diagnosis of Supplementary Learning Skills Inventoryshowed significant positive correlations between the Mixed construction of knowledge scale and the psychological factors of learning subscale (Table 7). The total score in the Diagnosis of Basic Learning Skills Task Battery also had significant positive correlations with the cognitive abilities subscale and the time management subscale of the Diagnosis of Supplementary Learning Skills Inventory.
Table 7
Correlations (Pearson correlation coefficient) between the Diagnosis of Basic Learning Skills Task Battery and the Diagnosis of Supplementary Learning Skills Inventory, with the general sample (N = 135)
|
Dependent construction of knowledge |
Independent construction of knowledge |
Mixed construction of knowledge
|
Total |
Motivation |
- |
- |
.195* |
|
Cognitive abilities |
- |
- |
.273** |
.237** |
Emotional state |
- |
- |
.193* |
|
Time management in the educational process |
- |
- |
.187* |
.183* |
Note. ** p < .01, * p < .05.
We used Multiple Regression Analysis (MRA) to specify the relationships between the scales of the two inventories. The differentiated parameters of each of the two inventories functioned as predictors of the other’s total score.
In the first regression model, we found a significant influence of the ability to perform Mixed construction of knowledge on the level of supplementary learning skills (Table 7.1). The other scales of the proposed modified method did not have a significant impact.
Table 7.1
First regression model for the scales of the Diagnosis of Basic Learning Skills Task Battery and subscales of the Diagnosis of Supplementary Learning Skills Inventory
Regression model |
Standardized values |
t |
Level of significance |
Beta |
|||
Constant |
36.558 |
22.340 |
.000 |
Mixed knowledge construction
|
.216 |
2.522 |
.013 |
The inverse regression model also showed a significant contribution of the cognitive abilities subscale in the development of basic learning skills (Table 7.2).
Table 7.2
Second regression model for the scales of the Diagnosis of Basic Learning Skills Task Battery and subscales of the Diagnosis of Supplementary Learning Skills Inventory
Regression model |
Standardized values |
t |
Level of significance |
Beta
|
|||
Constant |
17.061 |
6.532 |
.000 |
Cognitive abilities |
.237 |
2.778 |
.006 |
Differences in Basic Learning Skills Depending on the Stage of Learning
There were significant differences between the students from different stages of higher education in their basic learning skills, according to the proposed modified method. On average, students increased their basic learning skills (on all the scales) while proceeding through the learning stages (see Table 8). The most significant gain was in the Mixed construction of knowledge scale.
Table 8
Differences in the level of basic learning skills depending on the stage of learning (points)
|
First-year students |
Fourth-year students |
Second-year master students |
Significance of differences (Kruskal-Wallis H-test)
|
Dependent construction of knowledge
|
5.76 |
8.0 |
8.5 |
27.526 p = 0.000
|
Independent construction of knowledge |
4.8 |
6.2 |
7.2 |
29.261 p = 0.000
|
Mixed construction of knowledge
|
7.7 |
10.8 |
14.6 |
28.670 p = 0.000 |
N |
51 |
50 |
34 |
|
Discussion
Based on the analysis of the results, it can be argued that the modified battery is a valid and reliable tool for measuring basic learning skills. This is consistent with earlier findings that the level of basic learning skills is a predictor of academic performance of engineering students (ABET, Inc., 2013; Veenstra, Dey, & Herrin, 2008). The internal consistency between the tasks on the scales of the modified battery was acceptable.
Our research revealed the structure of basic learning skills for Russian engineering students; however, our Diagnosis of Basic Learning Skills Task Battery is suitable for use in all countries that adhere to Bologna system of education. Our results confirm earlier findings that general learning skills today play a valuable role in higher education (Hattie et al., 2013; Mamaril et al., 2016; Marra et al., 2017).
Correlational analysis showed significant correlations with psychological factors of learning for the Mixed construction of knowledge scale of the Diagnosis of Basic Learning Skills Task Battery. Multiple regression analysis revealed a significant effect of the ability to use Mixed construction of knowledge on the level of supplementary learning skills development. This is consistent with results that cognitive and mnemonic processes are linked with such psychological functions as motivation and will, emotions, and self-awareness (Gordeeva & Sichev, 2017; Hautamakiet al., 2002; Ilyasov, 2016; Raven & Stephenson, 2001).
Evaluation of the frequency distribution of students’ academic rating depending on their proficiency in basic learning skills revealed that the students whose average academic rating was close to the “satisfactory” mark demonstrated mostly low basic learning skills; students with a rating of “good” generally had average learning skills, while students with an average rating close to “excellent” showed average and high learning skills. This proved that the characteristics of academic success for engineering students include their learning skills (Veenstra, Dey, & Herrin, 2008).
The research showed significant differences in learning skills among the three stages of learning represented in the sample. So, as students went through various learning stages, their learning skills increased on all the scales, which is consistent with earlier findings that the process of basic learning skills development can be represented as the change and increasing sophistication of those skills throughout the learning process (Ilyasov & Kostrova, 2017).
Thus, our hypotheses were confirmed. In general, the results were consistent with the theoretical principles and demonstrated the structural validity of the Diagnosis of Basic Learning Skills Task Battery, which can be used to solve diagnostic problems.
Limitations
The research had some limitations. All participants were students from Moscow, and the number of participants was not equal in the different samples. In addition, the only criterion for validation at this stage is academic performance, in the absence of external validity of the test.
Acknowledgments
The authors are grateful to the administration of the Razumovsky University of Technology and Management, and personally to the director of the Institute of System Automation, Professor A.E. Popovich, Ph.D., for help with data collection, as well as to all the participants.
References
ABET, Inc., (2016). Criteria for accrediting engineering programs, 2016–2017. Retrieved from http://www.abet.org/accreditation/accreditation-criteria/criteria-for-accreditingengineering-programs-2016-2017/.
Butenko, V., Polunin K., Kotov I., Sycheva, E., Stepanenko, A., Zanina, E., & Topol’skaya, E. (2017). Russia 2025. From cadres to talents. The Boston Consulting Group (BCG). September 30, https://www.bcg.com/Images/Russia-2025-report-RUS_tcm27-188275.pdf (accessed: 04/23/2020).
Feuerstein, R., Rand, J., Hoffman, M., & Miller, R. (1980). Instrumental enrichment. An intervention program for cognitive modifiability. Baltimore, M.D.: Univ. Park Press.
Flavell, J.H. (1987). Speculations about the nature and development of metacognition. In F. Weinert & R. Kluwe (Eds.), Metacognition, motivation, and understanding (pp. 21–29) Hillsdale, NJ: Erlbaum.
Gordeeva, T.O., & Sichev, O.A. (2017). Motivatsionnye profili kak prediktory samoregulyatsii i akademicheskoi uspeshnosti studentov. [Motivational profiles as predictors of students’ self-regulation and academic achievement]. Vestnik Moskovskogo Universiteta. Seria 14. Psychologiya [Moscow University Psychology Bulletin], 1, 67–87 (in Russian, abstract in English). https://doi.org/10.11621/vsp.2017.01.69
Graf, V., Ilyasov, I.I., & Lyaudis, V.Ya. (1981) Osnovy samoorganizatsii uchebnoi deyatel’nosti i samostoyatel’naya rabota studentov [Fundamentals of self-organization of students’ educational activities and independent work]. Moscow: MSU.
Griffin, P. (2018). Self-regulated learning.Cambridge, UK: Cambridge University Press
Hattie, J., Biggs, J., & Purdie, N. (2013). Effects of learning skills interventions on student learning: A meta-analysis. Review of Educational Research, 66(2), 99–136. https://doi.org/10.3102/00346543066002099.
Hautamaki, J., Arinen, P., Eronen, S., Hautamaki, A., Kupiainen, S., Lindblom, …Scheinin, P. (2002). Assessing learning-to-learn: A framework. Helsinki, Finland: National Board of Education.
Ilyasov, I.I. (1986). Struktura protsessa ucheniya [The structure of the learning process]. Moscow: MSU.
Ilyasov, I.I. (2016). Rabota uchashchikhsya s faktorami ucheniya kak dopolnitel’nye uchebnye umeniya [Students’ work with learning factors as additional learning skills]. Zhurnal «Chelovek. Iskusstvo. Vselennaya»[Journal “Man. Art. Universe.”], 1,92–105 (in Russian, abstract in English)
Ilyasov, I.I., Malskaya, O.Ye., & Mozharovskiy, I.L. (1984). Formirovanie u studentov navykov uchebnoy raboty. Voprosy povysheniya kachestva podgotovki spetsialistov. [The formation of students’ learning skills. Issues of improving the quality of specialists’ training]. Moscow: MSU
Ilyasov, I.I., & Kostrova, A.V. (2017). Svyaz’ raznykh tipov ucheniya po P.Ya. Gal’perinu s vidami myshleniya uchashchikhsya [Connection between types of learning by P.Ya. Galperin with kinds of thinking in school students], Natsional’nyj psikhologicheskii zhurnal [National Psychological Journal], 3(27), 62–75 (in Russian, abstract in English). https://doi.org/10.11621/npj.2017.0308
Ilyasov, I.I., & Simonyan, M.S. (2018). Variant opisaniya sostava obshchikh uchebnykh umenii [Variant of describing the composition of general learning skills]. Materialy mezhdunarodnoi nauchnoi konferentsii «Deyatel’nostnyj podkhod k obrazovaniyu v tsifrovom obshchestve» [Abstracts of speeches from an international scientific conference, “The activity approach to education in a digital society, Moscow, Russia, pp. 153–158]
Khutorskoy, A.V. (2003). Klyuchevye kompetentsii kak komponent lichnostno-orientirovannogo obrazovaniya [Key competencies as a component of the personality-oriented educational paradigm]. Narodnoe obrazovanie [National Education],2,58–64.
Knapper, C. K., & Cropley, A. (2000). Lifelong learning in higher education(p. 238). London: Kogan Page.
Kraevsky, V.V., & Khutorskoy, A.V. (2003). Predmetnoe i obshchepredmetnoe v obrazovatelnykh standartakh [Subject and meta-subject in educational standards]. Pedagogika [Pedagogy],2,3–10.
Leontiev, D.A., & Klein, K.G. (2018). Kachestvo motivatsii i kachestvo perezhivanii kak kharakteristiki uchebnoi deyatel’nosti [The quality of motivation and the quality of experience as characteristics of learning activity]. Vestnik Moskovskogo Universiteta. Seria 14. Psykhologiya [Moscow University Psychology Bulletin], 4,106–119 (in Russian, abstract in English). https://doi.org/10.11621/vsp.2018.04.106
Lyaudis, V.YA., Ilyasov,I.I., Malskaya, O.E., & Mozharovskii, I.L. (Eds.).(1989). Formirovanie uchebnoi deyatel’nosti studentov[The formation of students’ educational activities]. Moscow: MSU.
Mamaril, N., Li, C., Usher, E.L., Economy, D.R., & Kennedy, M. (2016). Measuring undergraduate students’ engineering self-efficacy: A validation study. Journal of Engineering Education105(2), 366 –395. https://doi.org/10.1002/jee.20121
Marra, R.M., Kim, S.M., Plumb, C., Hacker, D.J., & Bossaller, S. (2017, June), Beyond the technical: Developing lifelong learning and metacognition for the engineering workplace. Paper presented at ASEE Annual Conference & Exposition, Columbus, OH, USA. Retrieved from https://peer.asee.org/27659
Mitrofanova, I.P., Simonyan, M.S. (2016). Razrabotka universalnoi modeli dlya proektirovaniya programm bakalavriata v vuzakh RF [Development of a universal model for designing bachelor’s programs in universities of the Russian Federation]. Mezhdunarodnyi nauchno-tekhnicheskii zhurnal «Teoriya, Praktika, Innovatsii» [International Scientific and Technical Journal “Theory, Practice, Innovation”],10(10), 4–12 (in Russian, abstract in English). Retrieved from http://tpinauka.ru/2016/10/Mitrofanova.pdf
National Academy of Sciences (NAS), Committee on Science, Engineering, and Public Policy (COSEPUP) (2005). Rising above the gathering storm: Energizing and employing America for a brighter economic future.Washington, DC: The National Academies Press.
Raven, J., & Stephenson, J. (2001). Competence in the learning society.New York: Lang.
Smerdon, E. T. (1996). Lifelong learning for engineers: Riding the whirlwind. American Ceramic Society Bulletin, 75,51–52.
Sternberg, R.J., & Williams, W.M. (2004). Does the Graduate Record Examination predict meaningful success in the graduate training of psychology? American Psychologist, 52,630–641. https://doi.org/10.1037/0003-066X.52.6.630.
Uden, L., & Dix, A. (2004). Lifelong learning for software engineers. International Journal of Continuing Engineering Education and Life-Long Learning, 14(2), 101–110. https://doi.org/10.1504/IJCEELL.2004.004578.
Veenstra, C. P., Dey, E. L., & Herrin, G. D. (2008). Is modeling of freshman engineering success different from modeling of non‐engineering success? Journal of Engineering Education, 97(4), 467–479. https://doi.org/10.1002/j.2168-9830.2008.tb00993.x.
Zimmerman, B. J. (2002). Becoming a self-regulated learner: An overview. Theory into Practice, 41(2),64–10. https://doi.org/10.1207/s15430421tip4102_2.
Appendix A
Example of a Task from the Dependent Scale of the ‘Diagnosis of Basic Learning Skills Task Battery’ for First-Year students
Read the passage and answer the question based on the text:
A trigger is the simplest memory element. All other nodes are based on triggers. Processor memory is usually divided into two essentially opposite types: internal (cache memory) and external (RAM). At the same time, the cache memory is absolutely static and has arbitrary access. Its main advantage is its speed. |
Question:
In this context, what property of RAM is present indirectly?
Describe it here:
Suggested answer: The author mentioned that the two types of memory are essentially opposite, which means that RAM is dynamic, with a complex access system and low performance.
Explanation of the answer: In the answer to this question, it is important to mention the dynamism of RAM, since the author talks about the type of “movement “ of cache memory, and also explains that the two types of memory are essentially opposite to each other.
Appendix B
Example of a Task from the Independent Scale of the ‘Diagnosis of Basic Learning Skills Task Battery’ for fourth-year students
To complete the task, you must remember the law of non-contradiction:
Two incompatible propositions cannot be simultaneously true, one of them must be false (it is not true that A and not A). |
Question:
In the technical wing of the University, the physics and computer science classrooms are located opposite each other. Fourth-year students decided to make fun of the first-year students and hung signs on the doors of classrooms:
“In one of these classrooms is the computer science room”, on the door of room A;
“The physics room is not located here”, on the door of room B.
The first-year students know that both inscriptions are either true or false. Provide a brief solution to the problem and fill in the table (“+”- Yes; “ - “ - no) in accordance with the correct location of the rooms.
| А | В |
The physics Room |
|
|
The computer science room |
|
|
Explanation of the answer:
If the sign on room A is correct, then:
| А | В |
The computer science room | + | + |
Logical equation: X = A | B (or)
If the sign on room B is correct, then:
| ¬A | ¬B |
The physics room | + | + |
Logical equation: Y = ¬А(not А)
Remember the law of non-contradiction:
(¬X¬Y) | (XY) = 1, that means ((A|B)*¬А)|((¬А|¬B)*¬¬A)=1 Simplify the equation step by step:
((A|B) * ¬А) = (A¬А)|(B¬А)
((¬А|¬B)*¬¬A)= ¬АA¬B
(A¬А) | (B¬А) = 0 | B¬А
¬АA¬B=0
Let’s go back to the original equation:
0 | B¬А = 1
B¬А = 1
Thus, B and not A are true; that is, a correctly filled out table will take the form:
| А | В |
The physics room | + | - |
| ¬А | ¬B |
The computer science room | - | + |
Appendix C
Example of a Task from the Mixed Construction Scale of the ‘Diagnosis of Basic Learning Skills Task Battery’ for Fourth-Year Courses
Read the passage and answer the question, whether the proposed conclusion is correct.
Any information system functions in an environment that is a source of input and a consumer of output information. Within such a system, from logging in to exiting, the information flow goes through several stages of processing. As you know, the main stages of information processing include the collection, registration, and primary processing of information, its transmission over the communication channel from the source to the PC, the creation and maintenance of information arrays, processing and formation of output forms, transmission over communication channels from computers to consumers, as well as conversion to a form convenient for the user. | ||
Conclusion: Information that enters the information system in one way or another forms an information array. | ||
a) The conclusion is correct | b) The conclusion is not correct | c) Insufficient information for the conclusion |
The expected answer: b) The conclusion is not correct.
Explanation of the answer:
The author touches on two main themes in the text: the relationship of information systems with the external environment and the stages of information processing.
The proposed conclusion does not directly affect either of them; however, information about the creation and maintenance of information arrays, as one of the stages of information transformation, is still present in the text. Therefore, we cannot say that there is not enough information for the proposed conclusion, and the proposed conclusion must be recognized as false.
To cite this article: Ilyasov, I.I., Aslanova, M.S., Bogacheva, N.V. (2020). ‘Diagnosis of Basic Learning Skills Task Battery’ Modified for Engineering Students. Psychology in Russia: State of the Art, 13(3), 145-160.
The journal content is licensed with CC BY-NC “Attribution-NonCommercial” Creative Commons license.